Part 2 of 4: Grow Revenue from Your Existing Customers: How Big Data Analytics Can Help
This post is the second in a four-part series. The first installment, “Go Beyond the Symptoms: How to Overcome Revenue Growth Challenges,” discussed the key signs of slowing growth and the first steps organizations can take to turn it around. Here, we’ll discuss three new ways in which companies can use Big Data analytics to improve a business’ ability to consistently improve revenue growth from their existing customers.
Big Data analytics in 2016 occupies roughly the same spot in the corporate consciousness as did the concept of cloud computing in 2008. By now, every world-class company that generates vast quantities of data has recognized that this data has exceptionally high value as an asset. These companies have made technology investments accordingly, procuring software solutions to organize, analyze, and manage the data, storage solutions (cloud or on-premise) to facilitate access to and distribution of the data, and often also professional services to enable and operate this infrastructure.
Meanwhile, data scientists continue to be a scarce and expensive resource. While universities are expanding their curricula to accommodate massive student demand for this as an academic discipline, it seems likely that supply will not be able to satiate demand for many years to come. Given this persistent shortfall, businesses must lean heavily on their existing data scientists to do more with the same resources, forge tighter collaboration between their data scientists and analytics teams that directly support the business functions, and rely on Big Data analytics solutions to provide the depth of capabilities and expanded capacity required.
New Possibilities for Customer Segmentation
As businesses strive to grow revenues from their existing customers, they confront the question of how best to apply Big Data analytics to solve this challenge. Segmentation clearly plays a key role in enabling customer analytics, but the traditional approach of starting with predefined segments may obscure key details, create groupings that don’t reflect reality, and cause analysts to draw inaccurate conclusions based on incomplete information or inferences that more quantitatively rigorous analysis doesn’t support. Big Data analytics can actually help the business derive customer segments based on patterns within the data.
Pattern-based groupings may be substantially different than the business’ prevailing segments, and these analytics-derived segments may not remain static over time. By letting the data itself guide the segmentation, the business can validate, refute, or modify its previous assumptions about the composition of its customer base. Additionally, deriving segments in this way exposes micro-segments that the business may have overlooked previously.
Understanding the attributes of customer segments is the necessary next step in using Big Data analytics to solve the revenue growth challenge. Traditional segmentation analysis proceeds to define descriptive attributes of each segment, and Big Data analytics can make this analysis faster, more comprehensive, and more accurate. The enhanced comprehensiveness and accuracy are a result of being able to go deeper into the data, identifying drivers of attributes and uncovering interdependencies and correlations that traditional analytical approaches miss entirely. For example, the business can reveal behavior patterns and driving factors not only for a particular customer micro-segment but also for individual customers within that segment, including patterns and drivers describing how specific individuals interact with other individuals. Additionally, this approach reflects the dynamic nature of customer attribute data and incorporates that dynamism into the analysis. As attributes change over time, the segmentation changes in tandem.
Customer Needs: Let the Data Do the Heavy Lifting
While traditional analysis then seeks to define customer needs that align with these attributes, Big Data analytics accelerates and enriches the analytical outcomes by more rigorously identifying and validating customer needs. These needs include those of which the customer is aware, as well as those generally subconscious “unknown needs” that also motivate purchase decisions. Smarter approaches to Big Data analytics go even further by defining “need states” — dynamic sets of behaviors, motivations, trade-offs, and thresholds — that guide purchasing behavior.
By identifying and comparing attribute and behavior patterns among customer groups and individual customers within those groups, the business can synthesize a rich data picture of its customers’ needs and need states. This data picture represents an entirely new level of predictive customer intelligence and allows the business to make more sophisticated and accurate decisions to drive greater revenue from those customers.
This combination of greater depth of insight, dynamism of attribute data, as well as objective derivation of segments, attributes, needs, and need states makes segmentation based on Big Data analytics the superior alternative to traditional approaches to segmentation and needs definition. As these parameters shift over time, the segmentation and needs definition shift with them, allowing the business to maintain a current view of the customer base and accurate predictive insight into customers’ responses to sales promotions, incentives, and other overtures. A business that takes this approach does not presuppose any outcomes of the segmentation and needs analysis, leading to more objective, accurate, and insightful analytical results, as well as stronger business results.
Streamlined Analytics Workflows that Extend to the Business Decision Maker
The analytical workflow itself is another key success factor for smarter Big Data analytics. Most businesses don’t utilize a single analytics solution but instead rely on multiple tools and technologies to develop and analyze a data picture of their customers. Extracting meaningful insights from that data picture and then converting that intelligence into a form that drives tangible business action involve both additional tools and extensive manual effort. The complexity and manual intensity of this effort elongate analytics cycle times and constrain throughput, limiting the value that Big Data analytics can deliver to the business. To rectify this technical and workflow challenge, businesses can consolidate their analytics activities on a single, highly capable Big Data analytics platform that efficiently manages the end-to-end workflow, enables agile testing and learning, and promotes scalability.
Finally, businesses seeking to maximize the contribution of their Big Data analytics efforts to revenue growth should engage the entire business, not merely the data scientists. After using data science to generate intelligence from their Big Data, businesses that infuse this intelligence directly into their enterprise applications and functional workflows allow business practitioners to readily take action based on this highly sophisticated, highly synthesized information. In this way, businesses can “democratize” Big Data analytics across the organization. Extending the power of data science throughout the organization in this way serves as a significant and sustainable “force multiplier” for value creation.
This combined approach to smarter Big Data analytics can lead to vastly better outcomes than traditional analysis and has powerful implications for efforts to grow revenue from existing customers.
John Mack is Executive Vice President of Marketing for Opera Solutions.
Part 2 of a 4-part series: “Grow Revenue from Your Existing Customers: How Big Data Analytics Can Help”
To learn more, view a recorded webinar that explores this topic in more detail, examines new ways to apply Big Data analytics to the challenge, and reviews case studies from industry-leading companies.
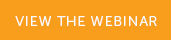